Summary :: MRI-based Brain Tumor Classification using Transfer Learning: A Comparative Analysis
The accurate and timely diagnosis of brain tumors is critical for patient outcomes, yet traditional methods like MRIs and biopsies often…
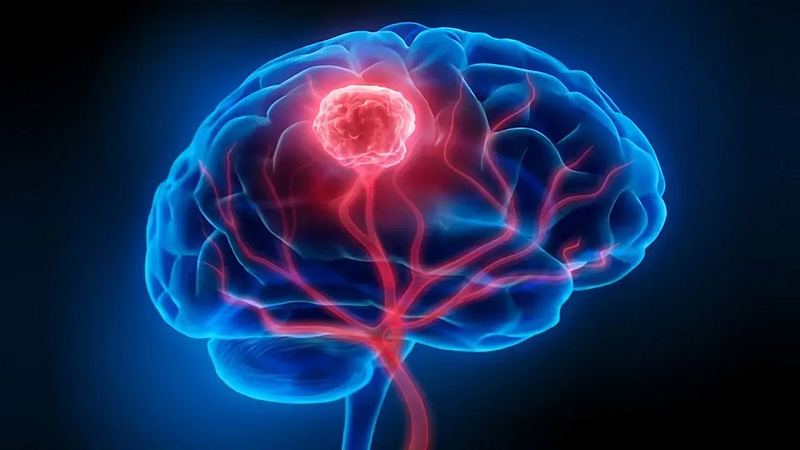
The accurate and timely diagnosis of brain tumors is critical for patient outcomes, yet traditional methods like MRIs and biopsies often lack consistency due to human error. This research, published in the International Journal of Computer Applications, evaluates the performance of five popular pre-trained models — VGG16, ResNet50, DenseNet121, InceptionResNetV2, and InceptionV3 — for classifying brain tumors from MRI images using transfer learning.
The study used a dataset of 7,023 MRI images, categorizing them into glioma, meningioma, pituitary tumor, and no tumor. DenseNet121 emerged as the top-performing model, achieving a validation accuracy of 94%, along with high precision, recall, and F1-scores of 0.94. Its performance surpassed the other models, making it the most efficient for brain tumor classification in this experiment.
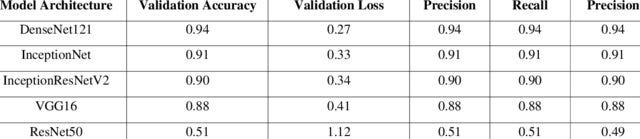
The study highlights the potential of transfer learning to significantly improve the accuracy and efficiency of medical image analysis. These findings point toward a future where AI and deep learning could enhance diagnostic precision, reduce human error, and improve access to healthcare in economically disadvantaged regions.
Read the full paper here: MRI-based Brain Tumor Classification using Transfer Learning: A Comparative Analysis