How IPL Clubs Use Data Science to Gain a Competitive Edge
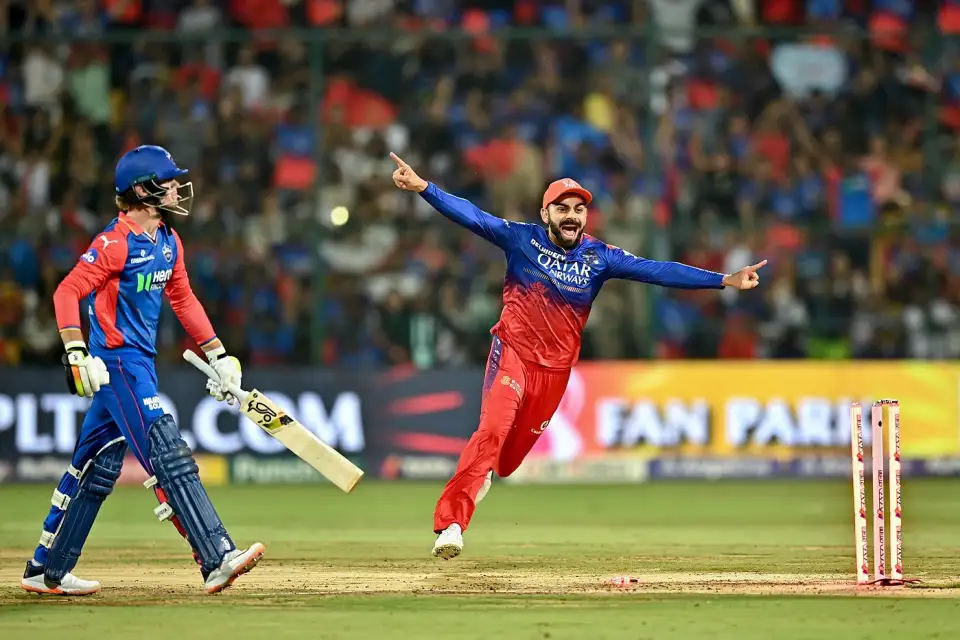
The Indian Premier League (IPL) is not just about big hits and thrilling finishes—it’s also a game of numbers. With millions of dollars at stake, IPL teams have turned to data science to gain a competitive advantage. From player selection to in-game strategies, franchises use advanced analytics to make informed decisions.
In this article, we explore how IPL clubs use data science to enhance their performance, scout talent, and maximize their chances of winning.
1. Player Performance Analysis
IPL franchises use data science to analyze player performance in domestic and international cricket before signing them in the auction. By examining:
- Batting Strike Rates against different types of bowlers
- Bowling Economy Rates in powerplays vs. death overs
- Fielding Impact Metrics like catches per match
Teams can determine which players will perform well under pressure and in specific match conditions.
Example: Rajasthan Royals were early adopters of data analytics, using it to scout lesser-known players like Sanju Samson, who later became a top performer.
2. Auction Strategy & Team Selection
The IPL auction is a high-stakes event where franchises bid for players. Data analytics helps teams identify undervalued players who can provide maximum impact at a lower cost.
- Predictive Analytics helps estimate a player's future performance based on past trends.
- Sentiment Analysis of social media and fan reactions gives insights into a player’s popularity.
- Injury Risk Assessment determines whether a player is prone to injuries.
Example: Moneyball-style analytics helped Punjab Kings invest wisely in young, cost-effective talent instead of overspending on big names.
3. Match Strategy & Game Planning
Coaches and analysts use real-time data to make better decisions during a match.
- Opponent Weakness Analysis: Data from past matches helps understand rival teams' vulnerabilities.
- Pitch & Weather Conditions: AI-driven models predict how a pitch will behave, helping captains decide whether to bat or bowl first.
- Bowling Matchups: Machine learning suggests the best bowler to use against a specific batsman based on historical data.
Example: Chennai Super Kings use match data to decide their batting order, often sending in specific batsmen against certain bowlers.
4. Fan Engagement & Marketing
Data science isn’t just for on-field performance—it also helps IPL franchises engage with fans.
- Social Media Analytics determines what type of content fans prefer.
- Personalized Marketing targets fans with merchandise and ticket offers based on their interests.
- AI-powered Chatbots enhance fan interactions by providing real-time match stats.
Example: Mumbai Indians use data analytics to optimize their social media engagement, ensuring higher fan interaction.
5. Injury Prevention & Fitness Management
Using biomechanical data and wearable technology, IPL teams monitor player fitness to prevent injuries.
- GPS Trackers & Sensors analyze workload and suggest rest periods.
- AI-Based Injury Prediction Models alert trainers before a player gets injured.
- Diet & Recovery Data help maintain peak performance throughout the tournament.
Example: Royal Challengers Bangalore use real-time fitness data to manage player workload efficiently.
Conclusion
Data science has transformed the IPL into a more strategic and analytical competition. From scouting talent and managing auctions to optimizing game strategies and engaging fans, every aspect of the IPL is driven by data.
As technology advances, we can expect IPL teams to rely even more on AI, machine learning, and predictive analytics to gain a competitive edge. In the future, real-time decision-making powered by AI could redefine how teams approach T20 cricket.